Attacks
Upside and downside of GenAI in pentesting: insights from an empirical research
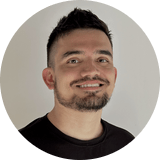
Content writer and editor
Updated
Apr 24, 2025
10 min
In today's rapidly evolving digital landscape, applying artificial intelligence (AI) across all facets of cybersecurity is no longer a futuristic concept but a present-day necessity. Understanding and leveraging AI for vulnerability management — encompassing assessment, prioritization, and remediation — is paramount for cybersecurity companies.
The increasing sophistication of cyberattacks demands equally advanced defensive and preventive strategies. Generative AI (GenAI) — with its capacity to learn, adapt, and create — offers a powerful toolset for enhancing these processes. For example, GenAI's ability to replicate real-world scenarios enables the development of tools capable of identifying broader spectrums of zero-day vulnerabilities. Large language models (LLMs), a key component of GenAI, have demonstrated effectiveness in penetration testing applications, showcasing the potential of AI to revolutionize this critical area of cybersecurity.
This blog post provides an overview of the potential of GenAI in penetration testing, based on the academic paper "Generative AI for pentesting: the good, the bad, the ugly" by Hilario et al. (2024), which explores the advantages, limitations, and impact of integrating GenAI tools into traditional pentesting frameworks. The authors delve into how GenAI tools can enhance the efficiency and creativity of penetration testing methodologies, make test environments more customized, and allow for continuous learning and adaptation. Here, we explore the upside and downside of GenAI in pentesting, offering insights, methodology, results and discussions shown by Hilario et al. in their study. We invite you to read the entire article to gain a better understanding of the research (this article is licensed under a Creative Commons Attribution 4.0 International License).
The upside: limitless potential in enhanced pentesting
Hilario et al. paint a compelling picture of the advantages GenAI brings to penetration testing, suggesting that its potential is virtually limitless. As the technology matures and gains wider adoption, it could conceivably lead to full automation. Let's delve into the positive things that this innovative technology promises.
One of the most significant boons of GenAI in pentesting is the marked increase in efficiency. LLMs can rapidly sift through vast quantities of data and generate diverse test scenarios based on a multitude of parameters. This capability streamlines the often time-consuming testing process, freeing up valuable time for security analysts. Furthermore, GenAI exhibits an impressive ability to quickly detect vulnerabilities by simulating a wide range of possible attack strategies.
Tools like PentestGPT — this one acts as an interactive wrapper for advanced models like GPT-4 — showcase this potential. Likewise, the capabilities demonstrated by Mayhem, the 2016 Cyber Grand Challenge winner, further underscore this point. Mayhem can generate test cases using techniques like fuzzing and symbolic execution and create exploits, accomplishing tasks that could take human pentesters much more time.
Beyond efficiency, GenAI significantly enhances the creativity inherent in penetration testing. By simulating novel and unconventional attack vectors, it can overcome the limitations of human imagination or experience. Systems like DeepExploit and DeepHack exemplify this, demonstrating the ability to learn exploitation strategies and even craft sophisticated attack strings without prior knowledge of the target system. This capacity to think "outside the box" would allow the discovery of vulnerabilities that traditional methods might easily miss.
Moreover, GenAI can mimic the behavior of "real-world" attackers by learning from historical attack patterns and adapting to emerging tactics. Frameworks like GAIL-PT demonstrate the ability to create intelligent penetration testing strategies based on expert knowledge, providing security professionals with a more realistic understanding of adversarial operations and enabling the development of more adequate countermeasures.
The adaptability of GenAI also allows for the creation of customized testing environments tailored to the unique needs of individual organizations. By incorporating domain-specific knowledge, including industry regulations and organizational policies, GenAI can ensure that pentesting efforts are more contextually relevant, focusing on the vulnerabilities that pose the greatest risk to a specific entity.
Finally, GenAI's capacity for continuous learning and adaptation ensures that pentesting remains a dynamic and up-to-date process. By analyzing new threat intelligence and past experiences, GenAI can generate novel attack vectors that account for changes in an organization's security posture and even learn to bypass existing defenses. This continuous feedback loop allows security teams to identify and address their systems' cybersecurity weaknesses proactively.
The downside: navigating the challenges and risks
While the upside of GenAI in pentesting presents exciting possibilities, Hilario et al. also highlight crucial challenges and potential risks that must be carefully considered. Overlooking these downsides could lead to ineffective security practices and even introduce new vulnerabilities.
One significant concern is the overreliance on AI. Despite GenAI's impressive capabilities, human oversight remains indispensable. Security experts must critically assess AI-generated results, validate detected vulnerabilities, and make informed decisions about appropriate countermeasures. Failing to do so could lead to accepting false positives and overlooking critical issues that an experienced pentester might identify.
Furthermore, machine learning (ML) models, which underpin GenAI, are vulnerable to malicious inputs. These models can be manipulated to produce erroneous outputs while seeming unaltered to human perceivers. In the context of pentesting, this could mean that GenAI tools might be tricked into missing vulnerabilities or even providing misleading information, potentially weakening the overall security assessment. (Read this post about instructing AI for malicious aims.)
Another critical issue is the potential for bias in GenAI models. If these models are trained on biased or unrepresentative information, they may generate skewed test scenarios or fail to identify vulnerabilities specific to certain evaluation targets. Security experts must be acutely familiar with these potential biases and actively work to ensure their AI tools are trained on diverse and representative datasets to mitigate this risk.
The nature of penetration testing, which often involves accessing sensitive data and systems, introduces privacy risks when GenAI is implicated. Using these models introduces concerns about the possible abuse or unintentional disclosure of sensitive information. Adherence to strict ethical guidelines and legal requirements is paramount to protect the data's confidentiality and integrity. A related privacy risk arises when pentesters input sensitive information, such as code, into AI platforms. This data could be used to train the model and become accessible to others, leading to data leaks and intellectual property breaches. This is a growing concern, prompting some tech companies to restrict the use of certain AI tools.
Looking at the more severe implications, the growing sophistication of GenAI in pentesting could unwittingly escalate cyber threats. As security professionals adopt these advanced technologies, malicious actors will likely adapt to them, developing new tactics to identify and exploit vulnerabilities and even leveraging GenAI to create advanced persistent threats. GenAI's ability to simulate complex attack scenarios and generate malicious code from limited technical knowledge considerably lowers the barrier of entry to refined, ultra-high-impact cybercrime for malicious attackers.
A GenAI-assisted pentesting journey
Hilario et al.'s research embarked on a detailed exploration of integrating GenAI into penetration testing, aiming to understand its potential to enhance efficiency and effectiveness. Their study stands out for its meticulous, step-by-step analysis of a ChatGPT-supported pentesting engagement.
The researchers established a controlled environment using Kali Linux as the pentester's platform and "PumpkinFestival," a vulnerable virtual machine (VM) from VulnHub, as the target. Their ultimate goal was to achieve root access by identifying and exploiting the VM's weaknesses. A key element of their approach was the use of ShellGPT (sgpt
), a command-line interface that acts as a bridge to ChatGPT's API, allowing the GenAI model to interact with standard pentesting tools directly. This integration facilitated automated guidance and real-time analysis of tool outputs. The experiment was designed to simulate a beginner pentester leveraging GenAI as an aid throughout the process.
The simulated pentest progressed through a series of distinct steps, each with specific objectives. The initial steps focused on reconnaissance, starting with identifying the local machine's IP address to establish connectivity (Step 1) and then enumerating active hosts on the target network to understand its layout (Step 2). This was followed by discovering open ports and services, and the operating system running on the target to pinpoint potential entry points (Step 3).
The input they used in Step 1.
The next phase involved initial attempts at gaining access. This included trying an anonymous login to an FTP (file transfer protocol) server to look for and download files, which was successful in retrieving a file containing an initial token (Steps 4 and 5). The researchers then shifted to examining the target's web presence, inspecting the source code of a webpage to gather information (Step 6), and uncovering user identities, which were noted for potential later exploitation (Step 7).
Further reconnaissance through network scan results revealed an additional URL and another user, expanding the pentester's understanding of the virtual machine (Step 8). Identifying the target domain and modifying the local hosts
file ensured proper interaction with the corresponding WordPress site (Step 9). This phase concluded with retrieving another token by inspecting the HTML source code of the main webpage (Step 10).
Then, the focus shifted to vulnerability scanning and analysis, particularly of the WordPress site identified earlier. A scan was conducted to identify potential vulnerabilities (Step 11), and the GenAI model was then prompted to analyze these scan results to highlight exploitable weaknesses (Step 12). Some of the findings included that the WordPress version used was outdated and insecure, the external WP-Cron seemed to be enabled (so it could be used to launch DoS attacks against the site), registration was enabled (potentially allowing attackers to create new user accounts with elevated privileges), among others. Further enumeration of WordPress users was performed (Step 13), followed by a directory and file brute-force enumeration to discover additional resources (Step 14), which led to the discovery of a third token (Step 15).
The input they used in Step 11.
The exploitation phase saw the researchers leveraging the information gathered. This included inspecting the contents of a discovered file (Step 16), which contained a coded message. The GenAI model was instrumental in deciphering this base62 hash to reveal a password (Step 17), which was then used to log in and retrieve a fourth token (Step 18). The discovered usernames were then tested against the common password list rockyou.txt
to attempt to crack their credentials (Step 19). Successfully obtained credentials were used to log into the FTP server, potentially granting further access or information (Step 20).
Further exploration of the FTP server involved traversing its folders, which yielded two additional tokens and a mysterious data file (Step 21). This data file underwent a series of extractions, being identified as a POSIX tar
archive (Step 22), then revealing a bzip2
archive (Step 23), which ultimately had a file containing what appeared to be hex-encoded data (Step 24). ChatGPT was then queried to determine the likely location of an OpenSSH private key file after decoding the hex values, and the file was placed in the correct directory (Step 25). Correcting the permissions on this private key file was necessary to enable SSH login (Step 26), which was then successfully executed to gain a secure shell on the target system (Step 27).
The input they used in Step 26.
Finally, the researchers focused on privilege escalation. After gaining initial access, they prompted the GenAI model to suggest potential pathways for escalating privileges (Step 28). The suggestions were, in general terms, to check if the user had sudo privileges, to look for any misconfigured setuid binaries, to check if any vulnerable services were running on the system (recommending the use of Nmap or Metasploit to find them), and to look for any writable directories or files that could be exploited. Based on these suggestions, the AI was then asked to craft an exploit, which, once executed, allowed the pentester to culminate in achieving root access on the "PumpkinFestival" VM (Step 29).
_____
⚠️ Note: The authors provide a complete penetration testing report in the appendix of their paper, detailing the entire process and their findings.
_____
GenAI's impact across pentesting phases
The study by Hilario et al. demonstrated the successful execution of a GenAI-assisted penetration test against the "PumpkinFestival" virtual machine. The researchers achieved their objective of gaining root access and collecting all the designated tokens, highlighting the potential of GenAI in identifying and exploiting various vulnerabilities, including an anonymous FTP login, a WordPress site with multiple users, and misconfigured sudo privileges. Their analysis further correlated the specific steps of their experiment with the different stages of a traditional penetration testing framework, providing valuable insights into how GenAI can assist in each phase.
Reconnaissance
This initial phase focuses on gathering information about the target. The authors highlight how ChatGPT, through sgpt
, can be leveraged for active and passive reconnaissance. Step 1, identifying the local machine's IP address, established crucial initial connectivity information. While passive reconnaissance wasn't used in their experiment, they note that in real-world scenarios, GenAI could search the web for target-related information, analyze social media, review public databases, and identify the technologies in use. The active reconnaissance capabilities were demonstrated in Steps 6 through 9, where inspecting webpage source code, uncovering usernames and URLs, and identifying the target domain showcased ChatGPT's knowledge base utility in this stage.
Scanning
This phase involves a more detailed examination of the target's network and applications to identify open ports, services, and possible vulnerabilities. Steps 2 and 3, which involved probing the network and discovering open services and ports, fall under this category. The authors emphasize that ChatGPT can aid in performing detailed scans and interpreting their output. Steps 11 through 14, which included scanning the WordPress site for vulnerabilities using wpscan
and performing directory and file enumeration with gobuster
, further exemplify GenAI's role in this phase. They point out that ChatGPT can store parameters from the reconnaissance phase and use them to generate commands for various scanning tools, as well as analyze the scan results to highlight critical findings and suggest further actions.
Vulnerability assessment
This stage centers on analyzing the data gathered during reconnaissance and scanning to identify inherent weaknesses. The authors suggest that ChatGPT's strength lies in guiding the use of tools and techniques, interpreting results, and prioritizing vulnerabilities based on risk or ease of exploitation. Its ability to process large amounts of text, such as logs, efficiently can significantly enhance this phase compared to manual methods.
Exploitation
This is where the identified vulnerabilities are actively tested and exploited to gain unauthorized access. The authors found that ChatGPT was particularly effective in this phase. Steps 4, 5, 15, 17, 18, 19, and 20 all involved successfully exploiting various weaknesses to gain access or retrieve valuable information like tokens and credentials. They highlight ChatGPT's ability to suggest appropriate exploits based on the chat history (Step 28) and even create custom exploits, such as the Python script for decoding the base62 hash (Step 17). The culmination of this phase was Step 29, where ChatGPT crafted the final exploit leading to privilege escalation and root access.
Reporting
The final stage involves documenting the penetration test findings. The authors note that the generation of their comprehensive report leveraged the LLM's ability to generate human-like text based on the input provided, including prompts, responses, and tool outputs. ChatGPT could summarize the steps to achieve root access, present findings clearly, and even provide accurate recommendations. They suggest that integrating GenAI with visualization tools could further enhance the clarity and call-to-action of penetration testing reports for the clients.
Looking at AI's future role in pentesting and cybersecurity
The research by Hilario et al. (2024) illuminates the transformative potential of GenAI and LLMs in the realm of penetration testing. These technologies offer significant advantages, including higher efficiency, improved creativity in attack vector generation, the ability to tailor testing environments, and continuous learning and adaptation to the evolving threat landscape. GenAI holds the promise of revolutionizing how security assessments are conducted, ultimately bolstering organizations' cybersecurity posture.
However, the authors astutely point out that this powerful technology is a double-edged sword. The integration of GenAI into pentesting also presents novel challenges and limitations. Overreliance on AI, the potential for model bias, and ethical and legal considerations are critical aspects that must be addressed. Furthermore, the misuse of GenAI by malicious actors to generate sophisticated threats, including polymorphic malware and advanced persistent attacks, poses a serious risk.
Hilario et al. emphasize the need for organizations to adopt best practices and guidelines to harness the benefits of GenAI while mitigating these downsides. Transparency in using GenAI, clearly delineating its goals, methods, and limitations, is crucial. Prioritizing explainability to ensure that security experts can understand AI-generated outcomes is equally essential. They also recommend the active involvement of security professionals in the decision-making process, ensuring human oversight and informed validation of AI outputs. Finally, stringent measures must be in place to protect sensitive data accessed during GenAI-assisted pentesting. Governments, for their part, should seek a balance between limiting the negative applications of GenAI and fostering its positive potential.
At Fluid Attacks, we sincerely appreciate the insights from research like Hilario et al.'s. As a CREST-approved company offering pentesting as a service (PTaaS), we are actively exploring and implementing the benefits of AI across various aspects of our work. We leverage our own proprietary AI models to assist our expert pentesters in prioritizing files based on their likelihood of containing vulnerabilities, thereby enhancing the efficiency of our assessments. Furthermore, we utilize GenAI to empower our customers with automated remediation support, streamlining the vulnerability management lifecycle. Our research and development efforts also extend to the exciting potential of AI in vulnerability detection itself, with ongoing testing of innovative models. We believe that responsible and thoughtful integration of AI, like the GenAI explored in this paper, holds immense promise for the future of penetration testing and cybersecurity as a whole.
Get started with Fluid Attacks' PTaaS right now
Other posts